By: Nicko Cajes; Northern Bukidnon State College, Philippines
Abstract
The continuous development of Internet of Things (IoT have exposed some important vulnerabilities, specifically in defending against the sophisticated DDoS attack. DDoS can successfully target IoT devices and make services unavailable which results in financial damages. With its huge potential threat, the necessity of developing an effective defense mechanism against it is needed. Existing defense mechanisms utilizing AI and proactive approaches such as rate limiting are one of the bright spots that aids in solving this issue.
Introduction
Through the last ten years, the Internet of Things (IoT) has evolved rapidly and also is projected to expand rapidly in the coming years because increasing numbers of fields search to obtain IoT-related solutions[1]. IoT is a complicated collection of interconnected devices known to transmit data through networks that are wireless or wired with the ultimate goal of providing assistance to individuals or technological devices [1, 2]. Due to factors like expenses, size, and power, safety administration has been neglected on the Internet of Things, it creates a major threat given that users become wary of utilizing IoT devices and if they are not secure, it will leave the network open to malicious activity and eventually results in substantial losses in both money and reputation [3]. This highlights the need for immediate action to evaluate current safety threats and talk about potential future threats so that we are prepared to handle them. This article will comprehensively discuss the future of IoT security against the sophistication of cyber-attacks, especially the distributed denial of services and on how to defend against it.
Understanding DDoS Attack and their Impact on IoT
DDoS attacks have a common life cycle and aim. To overwhelm the victim using fake traffic, the attacker dispatches commands on botnets which is a collection of compromised devices. DDoS aims to prevent genuine users from accessing servers [4]. Every internet action could suffer a long-term impact from this which in actual attack networks, there are far more devices [5]. This type of crime primarily aims to destroy systems, deplete their resources, and stop legitimate users on accessing its services. In the case of a DDoS attack, the targeted network is subjected to a denial-of-service attack by multiple attackers [6].
The rapid evolution and utilization of IoT in every field have gained the attention of the attackers, making it one of their targets to attack. IoT provides the ability to collect data gathered through electronic devices, draw conclusions from the data they produce, and continuously enhance such devices across multiple fields. Because IoT is diverse, it is vulnerable to a number of related security risks, such as compromises of privacy and integrity, an inadequate supply of resources, problems with reliability, etc. Due to security issues, the system is subject to many attacks, and the DDoS attack is becoming increasingly common among them [7].
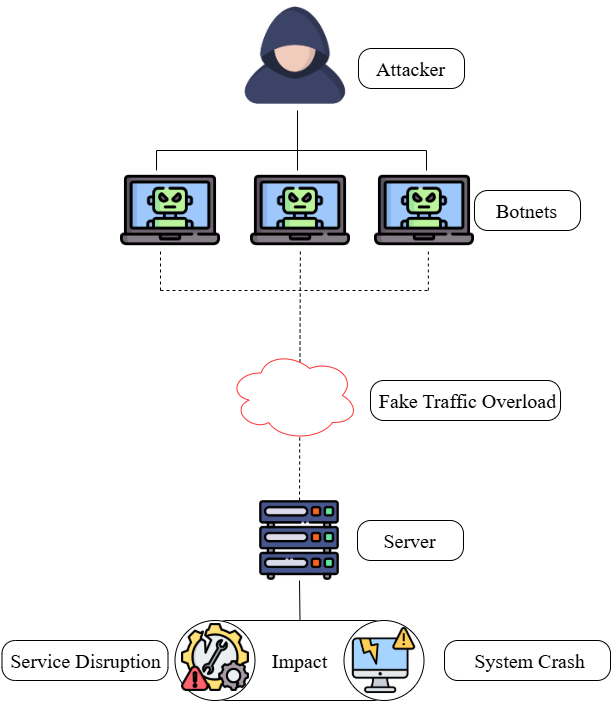
IoT Vulnerabilities Exposed by DDoS Attacks
Security concerns have emerged in the landscape of IoT, as its vulnerabilities have been exposed by DDoS attacks. The poor standard credentials on IoT devices are some of the main problems with security. Notable online attacks, such as the Mirai botnet, can enter through a security hole [8]. [9] enumerate the following reasons to highlight the importance of solving the common issues in IoT: First and foremost, when IoT devices are being used fraudulently, uncontrolled IoT systems do not simply endanger the security of users but may also have the potential to physically injure them. Second, they highlight a danger for producers, as businesses may lose important data and harm their brand once hackers gain possession of intellectual resources or private data via the Internet of Things system. Thirdly, the consequence of malicious activity extends across a single device or network because of the extensive level of interconnectedness of IoT systems. IoT network is just as safe as its most vulnerable link, hence the popular phrase that says in the chain, the strongest link is its weakest link is entirely true in this context.
A recently occurring and well-known instance of an actual DDoS attack is Mirai, which has previously resulted in significant harm and specifically took advantage of the inherent weaknesses of inadequately protected Internet of Things devices [10]. The primary challenge in countering this kind of attack is caused by the botnet’s ability to generate several authentic requests via HTTP that a system admin does not find untrustworthy [11]. The execution of collaborated attacks, in which multiple bot classes strike a single target under the direction of separate Command and Control (C&C) centers, represents an additional risky development of Mirai [12]. Making it quicker to send an enormous amount of traffic flow and making the server shut down as soon as possible.
IoT Vulnerability | Brief Description |
---|---|
Poor Credentials | Most IoT Devices have weak credentials |
Uncontrolled Characteristics | Compromised IoT Devices not just cause technological damage but physical damage as well |
Data Security | Confidential Data can be obtained using IoT networks |
Interconnectedness | IoT devices are linked with many devices making the widespread distribution of attack possible |
Attack Complexity | The use of botnet makes it difficult to detect malicious attack |
Table 1: IoT Vulnerabilities Exposed by DDoS
Strategies of Defending Against DDoS Attack in IoT
In order to defend and prevent being a victim of this sophisticated attack, strategies in defending against it have been already provided by a lot of researchers in the domain of cybersecurity. Strategies include the rate limitation, cand the use of AI specifically the Machine Learning based anomaly detection.
Rate Limitation: One common method for preventing network DDoS assaults is rate limitation, it is used to control the quantity of packets that are received when the rate of requests received grows too high for the server to manage, the rate limitation is frequently effective in preventing brute-force username and password attacks along with various forms of malicious activity aimed at the application or network layers [13].
Machine Learning-Based Anomaly Detection: Without any human involvement, machine learning-based anomaly detection techniques instantly recognize unusual trends of behavior in the attack dataset. Data concerning attack characteristics is used to develop machine learning techniques, the data gathered during training is used to create a model, and after that unusual trends or attacks in the real world are found using the model [14].
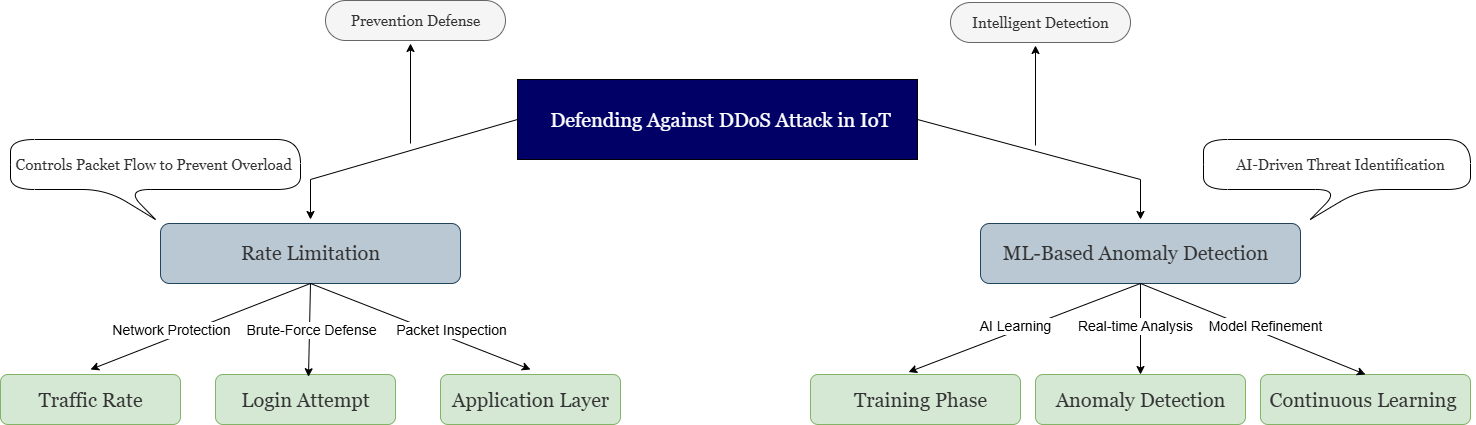
Conclusion
As the adoption of IoT becomes quick, the importance of addressing the vital vulnerabilities of IoT devices becomes a top priority. DDoS have posed a significant risk in this field, but emerging technologies utilizing ML have been one of the effective intermediaries against it. The continuous research on how to efficiently prevent being attacked and mitigate the attack are needed to ensure that IoT devices are going to be secure against this cyber-threat, especially in this modern age.
Reference
- Paolone, G., Iachetti, D., Paesani, R., Pilotti, F., Marinelli, M., & Di Felice, P. (2022). A holistic overview of the Internet of things ecosystem. IoT, 3(4), 398–434. https://doi.org/10.3390/iot3040022
- Rahaman, M., Lin, C. Y., Pappachan, P., Gupta, B. B., & Hsu, C. H. (2024). Privacy-centric AI and IoT solutions for smart rural farm monitoring and control. Sensors, 24(13), 4157.
- Mao, Y., You, C., Zhang, J., Huang, K., & Letaief, K. B. (2017). A survey on Mobile Edge Computing: The Communication Perspective. IEEE Communications Surveys & Tutorials, 19(4), 2322–2358. https://doi.org/10.1109/comst.2017.2745201
- Rahaman, M., Pappachan, P., Orozco, S. M., Bansal, S., & Arya, V. (2024). AI Safety and Security. In Challenges in Large Language Model Development and AI Ethics (pp. 354-383). IGI Global.
- Dong, K. Abbas and R Jain, “A survey on distributed denial of service (DDoS) attacks in SDN and cloud computing environments”, IEEE Access, vol. 7, pp. 80813-80828, 2019.
- S. D. Kotey, E. T. Tchao and J. D. Gadze, “On distributed denial of service current defense schemes”, Technologies, vol. 7, no. 1, 2019.
- Kumari, P., & Jain, A. K. (2023). A comprehensive study of DDoS attacks over IoT network and their countermeasures. Computers & Security, 127, 103096. https://doi.org/10.1016/j.cose.2023.103096
- Schiller, E., Aidoo, A., Fuhrer, J., Stahl, J., Ziörjen, M., & Stiller, B. (2022). Landscape of IoT security. Computer Science Review, 44, 100467. https://doi.org/10.1016/j.cosrev.2022.100467
- O. Garcia-Morchon, S. Kumar, M. Sethi, Internet of Things (IoT) Security: State of the Art and Challenges, Internet Research Task Force (IRTF), no. 8576, 2019, pp. 1–50,
- C. Kolias, G. Kambourakis, A. Stavrou and J. Voas, “DDoS in the IoT: Mirai and other botnets”, Computer, vol. 50, no. 7, pp. 80-84, 2017.
- Cirillo, M., Di Mauro, M., Matta, V., & Tambasco, M. (2021). Botnet identification in DDOS attacks with multiple emulation dictionaries. IEEE Transactions on Information Forensics and Security, 16, 3554–3569. https://doi.org/10.1109/tifs.2021.3082290
- A. Marzano et al., “The evolution of Bashlite and Mirai IoT botnets”, Proc. ISCC, pp. 813-818, Jun. 2018.
- Wang Fei, Hu Xiaofeng, Su Jinshu Unfair rate limiting for ddos mitigation based on traffic increasing patterns 2012 IEEE 14th International Conference on Communication Technology, Chengdu (2012), pp. 733-738
- Bhardwaj, A., Mangat, V., Vig, R., Halder, S., & Conti, M. (2020). Distributed denial of service attacks in cloud: State-of-the-art of scientific and commercial solutions. Computer Science Review, 39, 100332. https://doi.org/10.1016/j.cosrev.2020.100332
- Dahiya, A., & Gupta, B. B. (2021). A reputation score policy and Bayesian game theory based incentivized mechanism for DDoS attacks mitigation and cyber defense. Future Generation Computer Systems, 117, 193-204.
- Hammad, M., Abd El-Latif, A. A., Hussain, A., Abd El-Samie, F. E., Gupta, B. B., Ugail, H., & Sedik, A. (2022). Deep learning models for arrhythmia detection in IoT healthcare applications. Computers and Electrical Engineering, 100, 108011.
- Rahaman M. (2025) The Anatomy of a Smishing Attack: Common Techniques and Tactics Used by Cybercriminals, Insights2Techinfo, pp.1
Cite As
Cajes N. (2025) The Future of IoT Security: Defending Against DDoS Attack, Insights2Techinfo, pp.1