By: Vanna karthik; Vel Tech University, Chennai, India
Abstract
Internet of Things (IoT) devices have revolutionized multiple sectors through their spread which enables convenience features and offers automatic capabilities. The quick growth of IoT systems has produced major cybersecurity risks which artificial intelligence functions as both an advantage along with being a significant security threat. The use of AI by security defense systems exhibits increased protection measures but cybercriminals employ AI for advanced attacks against IoT devices. This paper examines the increasing threat from AI-controlled assaults against IoT systems while analyzing new attack pathways and specific incidents and explaining protection methods. The article explains the methods which cyber attackers utilize AI technology to execute automated reconnaissance while simultaneously exploiting system vulnerabilities and breaking standard security protocols. Artificial intelligence requires advanced defense tools that need proper regulations to combat the existing threats effectively.
Introduction
Modern society has accepted the Internet of Things (IoT) because it established effortless connectivity between smart home devices and industrial control systems. An expanding user base of Internet of Things drives the growing attraction of cybercriminals toward IoT systems. The implementation of AI has enabled attackers to build intricate methods for IoT network penetration which defeats traditional security methods making them obsolete[1]. Using AI to attack IoT devices ushered in a new security threat epoch which utilizes automation and machine learning together with deep learning to scan and breach multiple vulnerabilities on a massive scale.
The impact of Artificial Intelligence on IoT cyber-attacks during the present era
1. Automated Vulnerability Detection
Artificial Intelligence enables cybercriminals to search through extensive datasets so they can uncover weak points in IoT system infrastructure[2]. The application of AI-enabled hacking tools eliminates the need for manual work because these tools conduct quicker and more effective vulnerability exploration operations.
2. AI-Driven Malware and Botnets
Some malicious software types such as Mirai and Mozi prove how IoT devices can become part of botnets[3]. AI provides botnets with greater resistance since they constantly evolve their attack approaches to detect new security measures. Current antivirus programs fail to counter AI-powered malware because these systems both duplicate themselves independently and bypass traditional anti-malware protocols for improved resilience against detection.
3. Deepfake-Based Social Engineering Attacks
Deepfake technology enabled by AI produces realistic artificial content that allows criminals to hijack authentication systems as well as IoT operator controls and voice recognition functions[4]. Cybercriminals exploit the impersonation of valid users to breach unauthorized access points into smart devices and their corresponding networks.
4. Adversarial Machine Learning (AML) Attacks
The security systems implemented in IoT networks typically use AI-based anomaly detection technology. The security models which depend on machine learning encounter targeted assaults through Adversarial Machine Learning (AML) attacks since cybercriminals feed their models with counterfeit information to achieve undetected breaches[5].
5. Autonomous and Self-Learning Attacks
AI-driven cyber-attacks have started to function independently because they use security defenses as training material for endless evolutionary advancement. Trajectory adapted in real-time, so static security solutions prove useless for defending against such attacks[5].
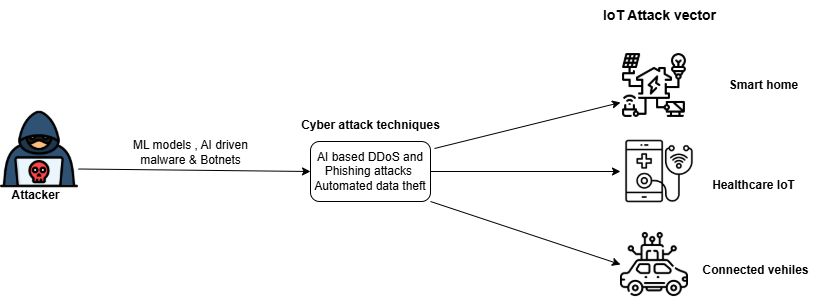
Real-World AI-Powered IoT Attacks [6]
The theoretical age of AI-powered cyber threats aimed at IoT devices has ended because they are now actively targeting this equipment. Various incidents demonstrate an increasing threat level to IoT systems.
The AI-enhanced botnet Mozi demonstrates self-teaching capabilities that use security patches to locate new vulnerability points in IoT devices.
Smart Home Devices Become Vulnerable Because AI-based brute force attacks breach smart locks together with security cameras and voice assistants to provide criminals with home entry.
AI-powered cyberattacks focus on critical infrastructure through Industrial IoT (IIoT) operations which lead to disrupted operations along with financial deterioration in manufacturing plants and power grids.
Mitigating AI-Powered IoT Attacks [7]
Professional security measures are essential for fighting AI-driven threats because organizations and individuals need to implement them.
1. Security solutions operated through AI technology must be implemented because they adapt automatically to emerging attack approaches.
2. Usage of Zero Trust Architecture implies strict security measures for authentication and access control which protects IoT network access points.
3. The proper application of updates and security patches to IoT devices through regular firmware deliveries enables the prevention of attacks by potential exploiters.
4. Behavioral Analytics together with Anomaly Detection systems utilize artificial intelligence security models to watch for abnormal device conduct which the system then detects immediately.
5. Manufacturers need to establish products with encryption protocols along with tamper-resistant features and secure system startup capabilities.
6. The authorities responsible for regulations should introduce rigorous cybersecurity guidelines for both IoT device manufacturers and end-to-end service providers.
Conclusion
A strong security threat emerges from AI-based IoT device attacks which presents cybersecurity challenges across all sectors. Security professionals must develop equivalent sophisticated defense mechanisms when attackers use AI to upgrade their cyber-attacks. Security protection based on artificial intelligence technology in addition to regulatory policies together with offensive security measures will defend against these developing security threats. The security of IoT-dependent systems will advance through persistent research together with teamwork and education effort to maintain superiority against adversaries powered by artificial intelligence.
References
- A. E. Omolara et al., “The internet of things security: A survey encompassing unexplored areas and new insights,” Comput. Secur., vol. 112, p. 102494, Jan. 2022, doi: 10.1016/j.cose.2021.102494.
- M. Kuzlu, C. Fair, and O. Guler, “Role of Artificial Intelligence in the Internet of Things (IoT) cybersecurity,” Discov. Internet Things, vol. 1, no. 1, p. 7, Feb. 2021, doi: 10.1007/s43926-020-00001-4.
- H. A. Almazarqi, A. K. Marnerides, T. Mursch, M. Woodyard, and D. Pezaros, “Profiling IoT Botnet Activity in the Wild,” in 2021 IEEE Global Communications Conference (GLOBECOM), Dec. 2021, pp. 1–6. doi: 10.1109/GLOBECOM46510.2021.9686012.
- M. S. Rana, M. N. Nobi, B. Murali, and A. H. Sung, “Deepfake Detection: A Systematic Literature Review,” IEEE Access, vol. 10, pp. 25494–25513, 2022, doi: 10.1109/ACCESS.2022.3154404.
- P. Haji Mirzaee, M. Shojafar, H. Cruickshank, and R. Tafazolli, “Smart Grid Security and Privacy: From Conventional to Machine Learning Issues (Threats and Countermeasures),” IEEE Access, vol. 10, pp. 52922–52954, 2022, doi: 10.1109/ACCESS.2022.3174259.
- T. N. Nguyen, Q.-D. Ngo, H.-T. Nguyen, and G. L. Nguyen, “An Advanced Computing Approach for IoT-Botnet Detection in Industrial Internet of Things,” IEEE Trans. Ind. Inform., vol. 18, no. 11, pp. 8298–8306, Nov. 2022, doi: 10.1109/TII.2022.3152814.
- P. Rahman and S. Mehnaz, “International Journal for Multidisciplinary Research (IJFMR),” SSRN Electron. J., 2024, doi: 10.2139/ssrn.5054029.
- Privacy-centric AI and IoT solutions for smart rural farm monitoring and control M Rahaman, CY Lin, P Pappachan, BB Gupta, CH Hsu – Sensors, 2024
- Gokasar, I., Pamucar, D., Deveci, M., Gupta, B. B., Martinez, L., & Castillo, O. (2023). Metaverse integration alternatives of connected autonomous vehicles with self-powered sensors using fuzzy decision making model. Information Sciences, 642, 119192.
- Zhou, Z., Li, Y., Li, J., Yu, K., Kou, G., Wang, M., & Gupta, B. B. (2022). Gan-siamese network for cross-domain vehicle re-identification in intelligent transport systems. IEEE transactions on network science and engineering, 10(5), 2779-2790.
- KEE S.N. (2024) Phishing Threats in IoT-Based Systems: Detection and Mitigation Techniques, Insights2Techinfo, pp. 1
Cite As
Karthik V. (2025) The Rise of AI Powered Attacks on IoT Devices, Insights2techinfo pp.1