By: Gonipalli Bharath, Vel Tech University, Chennai, India, & International Center for AI and Cyber Security Research and Innovations, Asia University, Taiwan, Gmail: gonipallibharath@gmail.com
Abstract:
Smishing, or SMS phishing, is an evolving cyber threat wherein attackers deceive people through text messages to steal sensitive information. The greater the use of mobiles, the greater is the scope for scams. This article discusses how Artificial Intelligence and Blockchain technology can be integrated to prevent smishing attacks. AI detects spoofed messages by using NLP and machine learning algorithms, and blockchain provides security to the communication by rendering the record decentralized and immutable. These technologies put together could bring about improved cybersecurity and prevent users from falling prey to smishing scams.
Introduction:
Due to rapid technology advance in mobile communication, smartphones are one of the major modes of communication. Smishing attacks take advantage of the dependency on smartphones by targeting smishing that leads users to reveal personal details such as bank details, passwords, and other confidential information[1]. The current conventional security practices are spam filters and user awareness, but these cannot cope with the evolving means of attacks. Therefore, AI and Blockchain need to be used to give a better security model for avoiding and reducing these scams[2].
Understanding Smishing Scams
Smishing scams usually come in the forms of fake SMS messages whereby fraudsters claim to be banks, government agencies, or other types of online services. In such messages, attackers send links to phishing sites or request confidential data. Hackers persuade users by using harmful hyperlinks, urgency-driven information, and psychological manipulation techniques.
Common Smishing Techniques:
- Fake Banking Alerts: Fraudsters send messages in the name of banks and ask users to verify their account details[3].
- Delivery Scams: Users get messages regarding the delivery of packages that contain malicious links[3].
- Fake Prize Notifications: Victims are enticed with prizes to fill out their personal details[3].
- Employment Scams: Messages related to offering jobs but actually redirecting them to some fraudulent portals[3].
Role of AI in Preventing Smishing
AI-powered techniques can analyze the text messages and detect patterns or flag suspicious content before it could reach the user. Following AI-driven approaches fortify the smishing detection:
- Natural Language Processing: NLP models can assess the message content and identify phishing language and abnormalities within the text patterns[4].
- Machine Learning Algorithms: Classification models, supervised and unsupervised, classify SMS messages as either legitimate or malicious based on the feature analysis of data like sender, message structure, URL patterns, etc.[5].
- Behavioral Analysis: AI keeps tabs on the users’ interaction with their environment, detects deviations from normal behavior, and triggers an alert when there is something suspicious regarding a given message.
- Real-Time Threat Detection: AI can process extensive data and use updated models regularly to detect emerging smishing techniques[6].
How Blockchain Helps in Combating Smishing
Blockchain is a decentralized way to enhance security for SMSs and prevent any unauthorized access. Here’s how it can help:
Immutable Record-Keeping – Blockchain keeps a tamper-proof record of the messages sent. It ensures full transparency and traceability.
Decentralized Authentication – It takes away the role of intermediaries and allows encrypted peer-to-peer communication, significantly reducing the potential for spoofed messages.
Verification by Smart Contracts – Blockchain will introduce smart contracts that will help verify the authenticity of messages sent and prevent malicious transmissions.
Public Key Infrastructure for Encryption – PKI provides encrypted messaging such that only those verified entities could send messages to users.
AI and Blockchain Integration for a Stronger Defence Mechanism
The integration of AI and Blockchain seems very promising in this fight against smishing scams. While AI models conduct analysis on incoming messages for fraud signals, Blockchain secures the fact that only verified senders get to transmit messages out, hence reducing the chances of smishing attacks.
Flowchart: Combining Artificial Intelligence and Blockchain to Prevent Smishing:
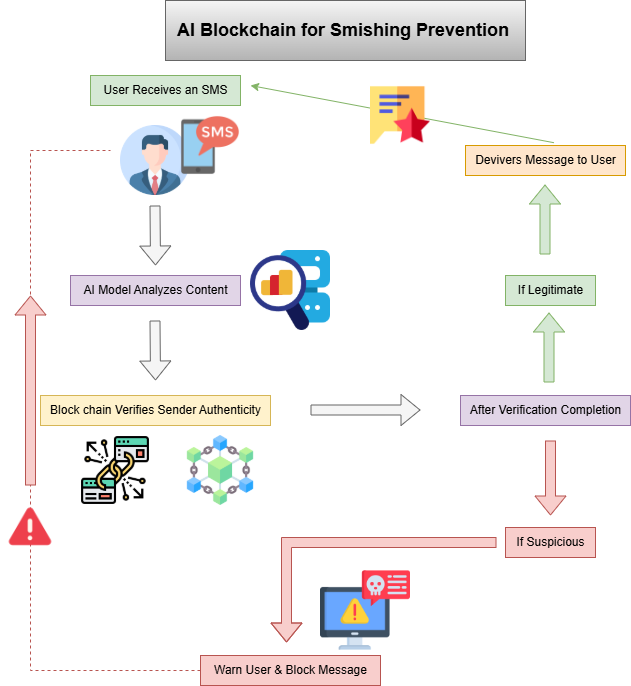
Fig(1)
Future Outlook: As cybercriminals continue to get better at their sinister games, so do the technologies of AI and Blockchain. Future developments could include:
- AI-powered chatbots for real-time scam detection.
- Blockchain-controlled identity verification in order to prevent unauthorized message transmission.
- Integration into mobile carriers for extended SMS filtering.
Conclusion:
Smishing incidents pose a major threat to the security of a mobile user by exploiting human psychology coupled with technological loopholes. Thus, AI and Blockchain are offering a powerful combat mechanism through scrutiny of SMS contents, authentication of senders, and securing of communication channels. Implementation of this will significantly lower smishing incidents and build up a safer digital environment.
References:
- E. Ustundag Soykan and M. Bagriyanik, “The Effect of SMiShing Attack on Security of Demand Response Programs,” Energies, vol. 13, no. 17, Art. no. 17, Jan. 2020, doi: 10.3390/en13174542.
- M. Rahaman, P. Pappachan, S. M. Orozco, S. Bansal, and V. Arya, “AI Safety and Security,” in Challenges in Large Language Model Development and AI Ethics, IGI Global Scientific Publishing, 2024, pp. 354–383. doi: 10.4018/979-8-3693-3860-5.ch011.
- M. L. Rahman, D. Timko, H. Wali, and A. Neupane, “Users Really Do Respond To Smishing,” in Proceedings of the Thirteenth ACM Conference on Data and Application Security and Privacy, in CODASPY ’23. New York, NY, USA: Association for Computing Machinery, Apr. 2023, pp. 49–60. doi: 10.1145/3577923.3583640.
- S. Sharma and T. Arjunan, “Natural Language Processing for Detecting Anomalies and Intrusions in Unstructured Cybersecurity Data,” Int. J. Inf. Cybersecurity, vol. 7, no. 12, Art. no. 12, Dec. 2023.
- A. Karim, S. Azam, B. Shanmugam, K. Kannoorpatti, and M. Alazab, “A Comprehensive Survey for Intelligent Spam Email Detection,” IEEE Access, vol. 7, pp. 168261–168295, 2019, doi: 10.1109/ACCESS.2019.2954791.
- M. R. A. Saidat, S. Y. Yerima, and K. Shaalan, “Advancements of SMS Spam Detection: A Comprehensive Survey of NLP and ML Techniques,” Procedia Comput. Sci., vol. 244, pp. 248–259, Jan. 2024, doi: 10.1016/j.procs.2024.10.198.
- Sedik, A., Hammad, M., Abd El-Samie, F. E., Gupta, B. B., & Abd El-Latif, A. A. (2022). Efficient deep learning approach for augmented detection of Coronavirus disease. Neural Computing and Applications, 1-18.
- Deveci, M., Pamucar, D., Gokasar, I., Köppen, M., & Gupta, B. B. (2022). Personal mobility in metaverse with autonomous vehicles using Q-rung orthopair fuzzy sets based OPA-RAFSI model. IEEE Transactions on Intelligent Transportation Systems, 24(12), 15642-15651.
- Lv, L., Wu, Z., Zhang, L., Gupta, B. B., & Tian, Z. (2022). An edge-AI based forecasting approach for improving smart microgrid efficiency. IEEE Transactions on Industrial Informatics, 18(11), 7946-7954.
- Navaneeth J. (2024 ) Blockchain-Enabled Security: Revolutionizing Data Protection, Insights2Techinfo, pp.1
Cite As
Bharath G. (2025) The Role of AI and Blockchain in Preventing Smishing Scams, Insights2Techinfo, pp.1