By: K. Sai Spoorthi, Department of computer science and engineering, Student of computer science and engineering, Madanapalle Institute of Technology and Science, 517325, Angallu, Andhra Pradesh.
Abstract
AI particularly generative AI is revolutionizing the way forecasting is done in the finance sector through providing adaptive models as opposed to the usual non generative models which rely on the past records. With the help of complex mathematical methods and large volumes of data, generative AI enhances the performance indicators that directly impact real-time analysis. Hence methods like Generative Adversarial Networks (GANs), Variational Autoencoders (VAEs) for the generation of synthetic data to help in better modelling and simulating the market to assess the risk. Thus, retail giants like Walmart reveal what competitive benefits and improved productivity the use of generative AI brings. Thus, while there are issues with data protection and model explanation, the impact of generative AI to transform the forecasting of financial performance is massive. Solid frameworks are needed to get most of the benefits and avoid the risks which makes generative AI one of the most valuable methods to achieve accurate and significant financial forecasts.
Key words: Generative Artificial Intelligence, financial forecasting, real-time data, sustainability, Generative Adversarial Networks (GANs), Variational Autoencoders (VAEs),
Introduction
It is also necessary to pay attention to the fact that the modern condition of the financial world is very interconnected with the issues of the speed of the technological advances. particularly generative artificial intelligence (AI), and such systems that are made to produce content and modelling complex situations offer optimistic opportunities to predict financial operations. Given the fact that markets are not stagnant and are even connected in the present-day world simple trend forecasting models are not only limited in terms of accuracy but also in terms of flexibility. However, there is Generative AI which uses big data and other enhanced algorithms to design relevant and dynamic models that could predict future data. In this essay, I am going to explain how, in my opinion, application of generative AI enhances financial forecasting, with the emphasis on such aspects as precision, working in real time, and the main decision maker during uncertainty.[1] In this case, therefore, by showing how the generative AI works through real-life illustrations and comparing these with the existing models, it should be possible to bring to light how this type of application can reform the notion of best practices when it comes to the finance sphere with a view to come up with sustainable models of investments and a sustainable economy.
Financial Forecasting and the Emergence of Generative AI
Budgeting and the process of financial forecasting in earlier times was entirely based on the statistical analysis of past trends and data of future financial performance. However, due to the current progression in Generative AI, these practices are set to be redefined through incoming forms of dynamic and adaptive forms of forecasting methods. Generative AI systems use algorithmic approaches and massive datasets, which allows considering numerous patterns in the market and integrate different indicators in real-time, which increases the accuracy of the models’ predictions. This shared transformation is same as the generative design in the built environment where superior technologies are helpful in getting imaginative and sustainable solutions. Thus, the integration of the concept can be useful for organizations that try to manage their existence in a fluctuating economic environment that not only helps to automate the processes of forecasting but also increases the ability of businesses to act efficiently to the emerging trends which are most relevant to the strategic plans of an organization[2]. Ultimately, the emergence of generative AI introduces a new wave of paradigm shifts in financial forecasting in terms of risk.
The Mechanisms of Generative AI in Financial Forecasting
Besides, generative AI systems can take the sophisticated data sets, develop their pattern and trending that are often beyond human ability and analysis. The common generative AI methodologies like the neural networks and decision trees helps in early generation of simulations of the actual financial condition and the possibility of occurrence of conditions based on historical patterns as well as current conditions. It is also useful for the predictive capability especially in volatile markets where the adjustments can be made faster affords a company a competitive advantage[3]. Moreover, such systems could acquire training over new data or improve on the existing models to improve the accuracy in the process bearing in mind that problems of outdated figures that characterizes static forecasting methods. The integration of generative AI in financial forecasting thus not only provides equal opportunities to use complex analysing tools to various organizations but also enables an adequate reaction to new shifting factors that influence economic environments
Techniques and Algorithms Used in Generative AI for Data Analysis
When it comes to fate casting in financial environments, generation AI techniques and algorithms have been largely developed, which creates a premise for the improvement of the prediction systems. Very important data handling strategies like Generative Adversarial Networks (GANs), Variational Autoencoders (VAEs), etc., are extremely useful for handling complicated datasets for the synthesis of artificial data very much resembling real data patterns. This synthetic data is important in developing machine learning models when and where the prediction of financial trends is highly accurate[4]. Also, algorithmic procedures are useful to consider different options regarding various situations that may occur in the market; thus, such strategies would be helpful for analysts as the markets are constantly evolving. Since generative design principles have the capability of transforming methodologies in built environments as mentioned above, in the same way, generative AI for data analysis can revolutionize the financial practices and bring out the best in existing or new methods of forecasting. Therefore, by incorporating effective algorithmic structures, the finance sector will be able to realize better predictive analytics as the application of generative models is steadily prevailing within this context.
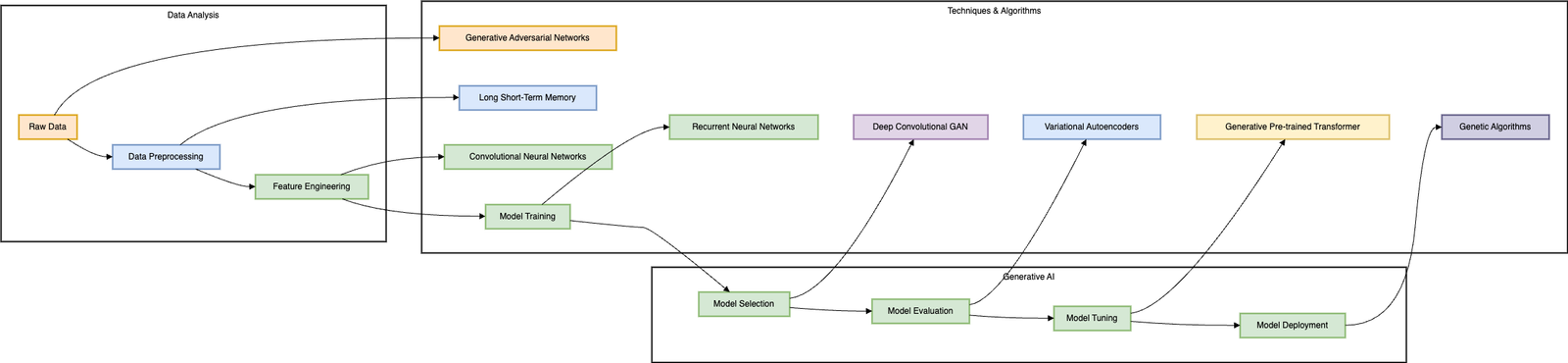
Figure 1 Generative AI Techniques
Applications and Case Studies of Generative AI in Financial Forecasting
Recently, machine learning and specifically generative AI has been used to expand and improve modern financial predictions, acquiring highly detailed relationships that can potentially generate improved options for forecasting the behaviour of the marketplace. One fascinating use is the combination of machine learning techniques with past records to create artificial data that firms can then use for different scenarios of the financial world. Such simulations enable the analysts to ascertain the effects that market fluctuations actually have on performance of assets, hence enabling knowledgeable investment. For instance, there are multiple examples of usage of generative models, including GANs, to strengthen modern risk evaluation approaches, which in turn affects the management of existing portfolios[5]. However, using these models they have noted that it can be applied to improve the organizations’ predictive modelling which in return helps in enhancing predictive analytics to a competitive level in terms of market and consumer trends. In this case, evolution of generative AI to this context means a shift from the regular financial seclusion in forecasting through more adaptive methodologies supported by data.[6]
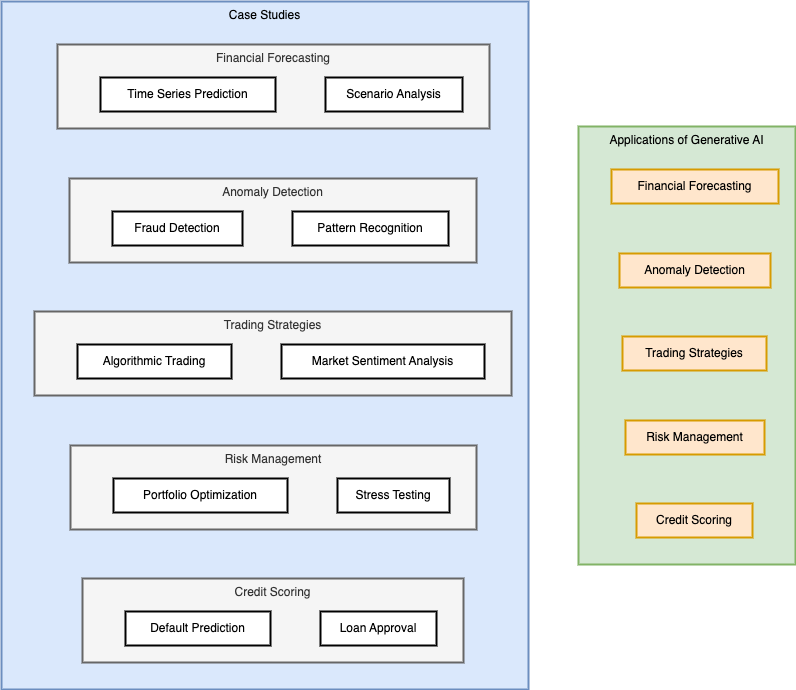
Real-World Examples of Generative AI Enhancing Predictive Accuracy
Deployment of generative AI in the creation of forecast for financial provisioning has recorded reasonable advancement in the aspect of prediction. across various industries. For instance, in retail where Walmart and other huge firms are still operating, generative models have been implemented to handle large data that may influence consumers’ actions and balance stocks. It is to state that this accuracy enhances the Organizational efficiency not only the customer satisfaction because of the product availability. however, through the generation of numerous market situations, the generative models aid the applied financial models in providing better information since they can generate various market situations to arrive at the best strategies that can be enacted. Thus, by implementing the application of generative AI in risk assessment activities, a better risk vision is attained in the process eventually minimizing the losses possible in the organization[7]. The more the firms harness these capabilities, they are offered an advantage, which discreetly shows the disrupting aspect of this technology regarding the projection of funds. Therefore, such advancements indicate for a further study of the uses of generative models which highlights its ability to generate for the future of financial analysing and businesses.
Conclusion
The adoption of Generative AI in the aspect of financial forecasting can be seen to offer new generation shifts in terms of innovation in the analysis functionalities and decisions. While organizations use these advanced models the chances of improving on the accuracy of predictions are apparent; though the management of the challenges that accompany them must be well tackled. The findings only suggest that Generative AI is not only useful in changing the theoretical constructs of supply chain management, but it is also versatile in other industries such as financial services. However, as discussed by threats ranging from information inaccuracy, lack of interpretability, and data privacy issues remain a menace to citizen data scientist as well as the overall growth of financial forecasting. Thus, for Generative AI to be and remain the game-changing tool, which it is recognized to be, financial institutions need to create solid frameworks that will unlock its possibilities while protecting against its dangers. It will help in development of the balanced approach that will let Generative AI become the reliable assistant in the process of obtaining the accurate and meaningful financial data.
References:
- T. Haksoro, A. S. Aisjah, Sreerakuvandana, M. Rahaman, and T. R. Biyanto, “Enhancing Techno Economic Efficiency of FTC Distillation Using Cloud-Based Stochastic Algorithm,” Int. J. Cloud Appl. Comput. IJCAC, vol. 13, no. 1, pp. 1–16, Jan. 2023, doi: 10.4018/IJCAC.332408.
- A. Lopez-Lira, The Predictive Edge: Outsmart the Market using Generative AI and ChatGPT in Financial Forecasting. John Wiley & Sons, 2024.
- X. Bai, S. Zhuang, H. Xie, and L. Guo, “Leveraging Generative Artificial Intelligence for Financial Market Trading Data Management and Prediction,” Jul. 01, 2024, Preprints: 2024070084. doi: 10.20944/preprints202407.0084.v1.
- R. K. Verma and N. Kumari, “Generative AI as a Tool for Enhancing Customer Relationship Management Automation and Personalization Techniques,” Int. J. Responsible Artif. Intell., vol. 13, no. 9, Art. no. 9, Sep. 2023.
- A. Selvaraj, C. Althati, and J. Perumalsamy, “Machine Learning Models for Intelligent Test Data Generation in Financial Technologies: Techniques, Tools, and Case Studies,” J. Artif. Intell. Res. Appl., vol. 4, no. 1, Art. no. 1, Feb. 2024.
- B. D. Alfia, A. Asroni, S. Riyadi, and M. Rahaman, “Development of Desktop-Based Employee Payroll: A Case Study on PT. Bio Pilar Utama,” Emerg. Inf. Sci. Technol., vol. 4, no. 2, Art. no. 2, Dec. 2023, doi: 10.18196/eist.v4i2.20732.
- P. Kumar et al., “AI-enhanced inventory and demand forecasting: Using AI to optimize inventory management and predict customer demand,” World J. Adv. Res. Rev., vol. 23, no. 1, Art. no. 1, 2024, doi: 10.30574/wjarr.2024.23.1.2173.
- Gupta, B. B., & Panigrahi, P. K. (2022). Analysis of the Role of Global Information Management in Advanced Decision Support Systems (DSS) for Sustainable Development. Journal of Global Information Management (JGIM), 31(2), 1-13.
- Gaurav, A., Gupta, B. B., & Panigrahi, P. K. (2022). A novel approach for DDoS attacks detection in COVID-19 scenario for small entrepreneurs. Technological Forecasting and Social Change, 177, 121554.
Cite As
Spoorthi K.S. (2024) The Role of Generative AI in Financial Forecasting, Insights2Techinfo, pp.1